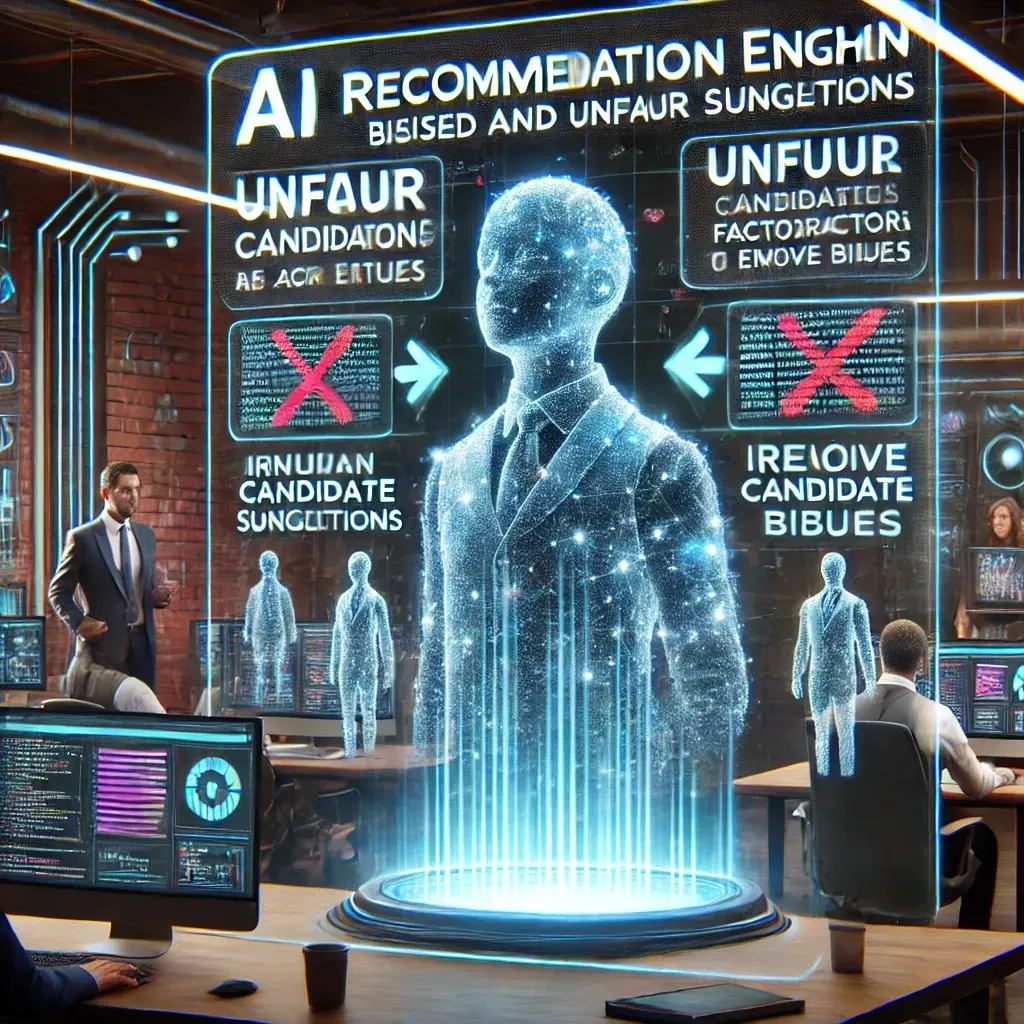
When AI Discriminates – Fixing a Broken Recommendation Engine
When AI Discriminates – Fixing a Broken Recommendation Engine
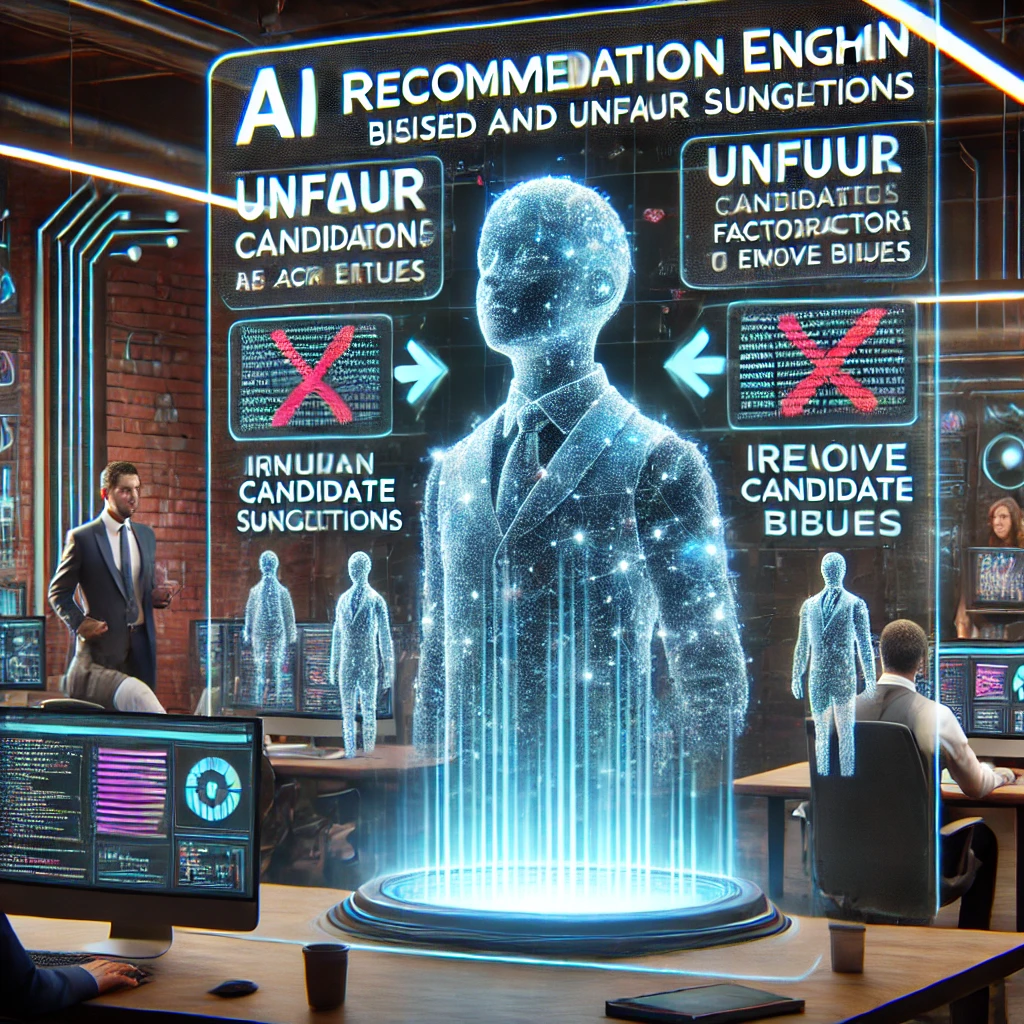
Introduction
AI is supposed to improve hiring decisions, but what happens when it gets it wrong? AI-driven recommendation engines—used for candidate screening, job matching, and performance predictions—can unintentionally perpetuate discrimination, exclude qualified talent, and damage employer trust.
But here’s the good news: AI bias is fixable. In this guide, we’ll break down how to spot, diagnose, and repair a biased AI hiring system before it starts costing you top candidates and compliance headaches.
Why AI Hiring Bias Happens
Before we fix the problem, let’s understand why AI sometimes makes biased decisions:
�� Biased Training Data – If past hiring data is skewed, AI learns and replicates those biases.
�� Overfitting to Patterns – AI might prioritize patterns that historically led to successful hires, even if they’re discriminatory.
�� Lack of Diversity in AI Design – AI models are often built by homogeneous teams, unintentionally overlooking bias risks.
�� Opaque Algorithms (‘Black Box’ AI) – If recruiters can’t understand why AI rejects candidates, they can’t correct unfair patterns.
Case Study: "A major tech company discovered its AI automatically downgraded resumes with women’s colleges, reflecting historical gender bias in engineering hires."
How to Spot AI Discrimination in Hiring
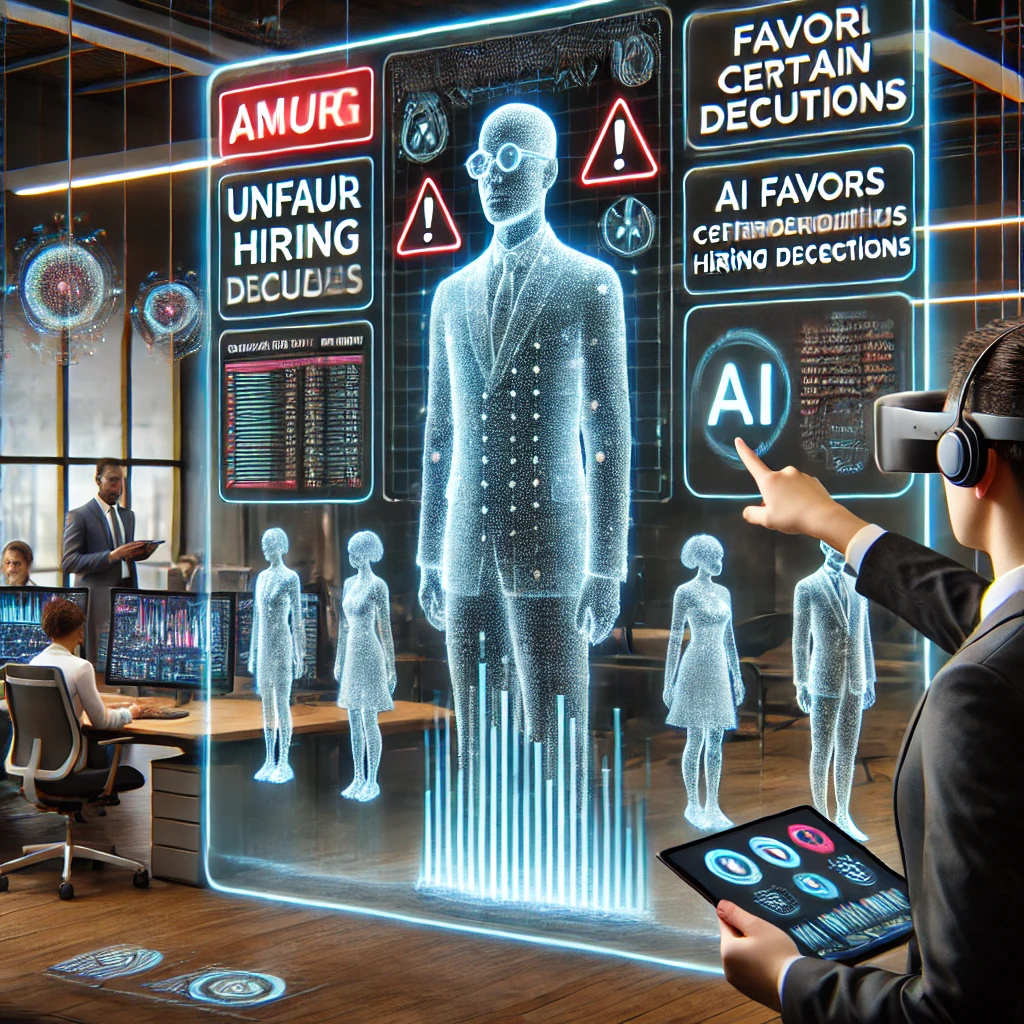
To fix AI bias, you first need to identify where it’s happening. Here’s how:
�� Audit AI Hiring Decisions – Compare AI-approved vs. rejected candidates by demographic breakdown.
�� Run Bias Simulations – Input diverse candidate profiles to test if AI systematically favors/disfavors certain groups.
�� Analyze Unintended Exclusions – Review candidate drop-off points to detect AI-driven filtering trends.
�� Track Candidate Feedback – If high-quality candidates complain about unfair rejection, investigate AI patterns.
Example: "An AI resume screener systematically deprioritized candidates with employment gaps—affecting returning parents and career changers disproportionately."
5 Ways to Fix a Biased AI Hiring System
Once bias is detected, it’s time to course-correct. Here’s how:
1. Retrain AI Models with Diverse Data
�� Expand training datasets to include a wider range of backgrounds, experiences, and industries.
�� Eliminate bias triggers—ensure AI doesn’t rely on irrelevant factors (e.g., names, ZIP codes, gender).
�� Normalize data inputs so AI doesn’t favor one demographic over another.
2. Adjust AI Decision-Making Weightings
⚖️ Review and rebalance AI scoring metrics—reduce over-reliance on traditional success indicators that skew against diversity.
⚖️ Introduce human-adjusted ‘fairness corrections’ to fine-tune AI scoring models.
⚖️ Use counterfactual fairness testing to ensure similar candidates get similar outcomes.
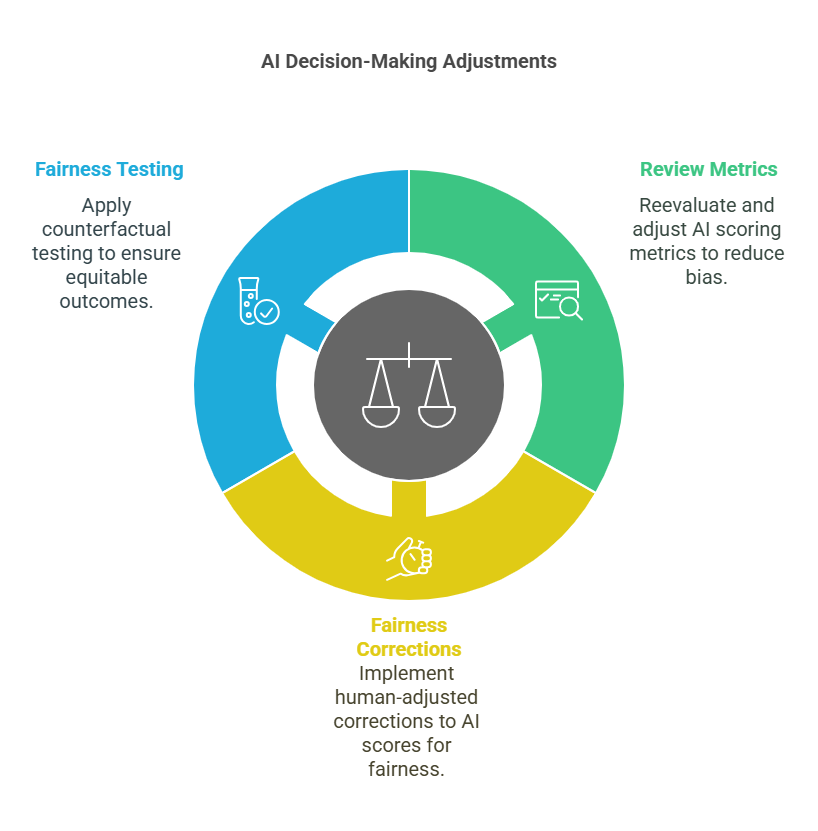
3. Increase Transparency & Explainability
�� Make AI decisions explainable—candidates and recruiters should understand why AI selected or rejected someone.
�� Enable human override options—let recruiters manually adjust AI decisions in questionable cases.
�� Regularly publish AI fairness reports—track hiring trends to identify any resurfacing bias.
4. Implement Bias Auditing & Compliance Checks
��️ Conduct third-party AI audits—external fairness reviews catch blind spots.
��️ Adopt AI bias-checking tools—use platforms that proactively detect discrimination (see tools below).
��️ Stay GDPR & EEOC Compliant—ensure AI hiring meets legal fairness standards.
5. Blend AI with Human Oversight
�� Never let AI make final hiring decisions alone—AI should assist recruiters, not replace them.
�� Encourage human-AI collaboration—recruiters should always review AI recommendations critically.
�� Educate hiring teams on AI bias risks—so they can intervene when necessary.
Example: "After implementing fairness adjustments, a hiring AI that previously favored Ivy League graduates saw a 22% increase in candidates from public universities being shortlisted."
Best AI Bias-Checking & Fair Hiring Tools
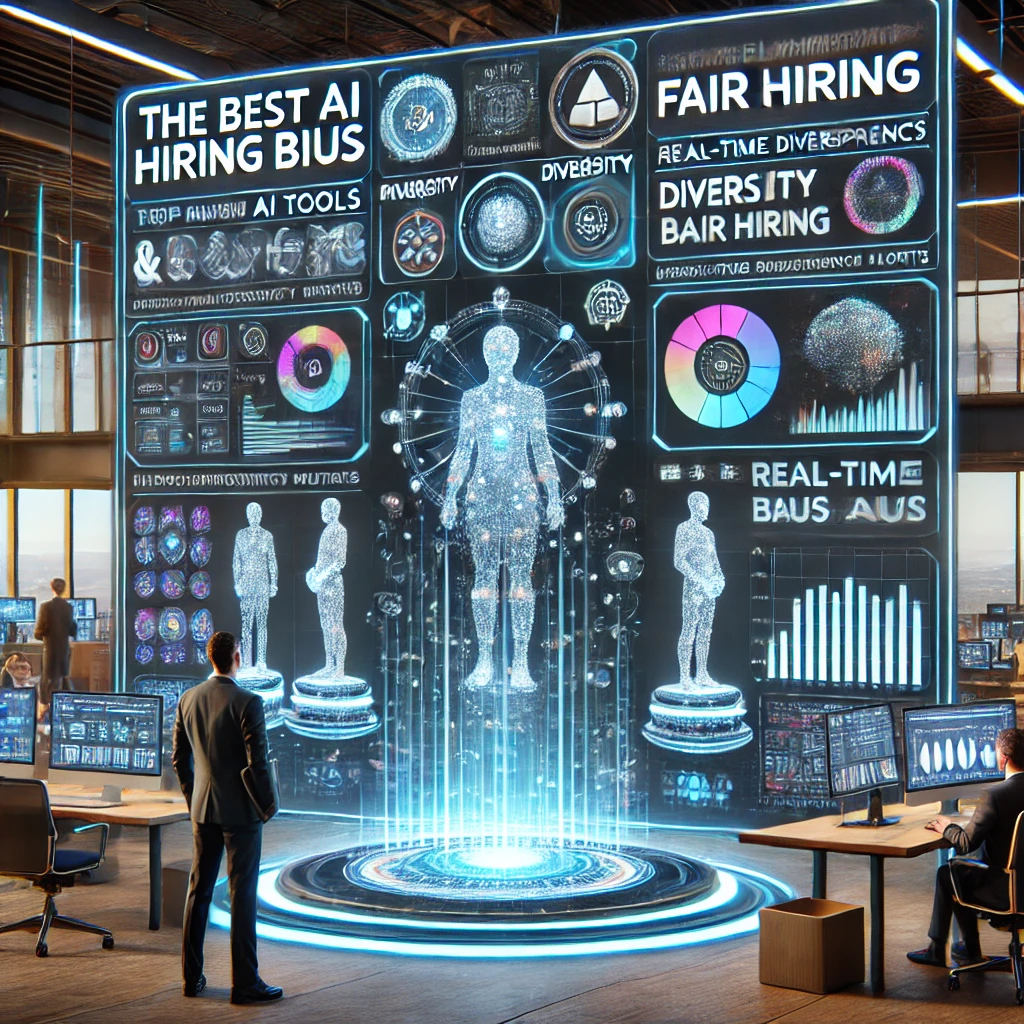
Want to prevent AI discrimination? These top AI fairness tools help detect and fix hiring bias:
�� IBM AI Fairness 360 – Bias detection and fairness scoring for AI models.
�� Pymetrics Audit AI – AI fairness audits for recruitment algorithms.
�� Textio Fairness Metrics – Analyzes job descriptions for biased language.
�� HiredScore Fair Hire Index – Real-time fair hiring compliance tracking.
Fun Fact: "Companies that actively monitor AI fairness see a 30% improvement in diversity hiring outcomes."
Common AI Bias Pitfalls & How to Avoid Them
Even well-intended AI systems can backfire. Here’s what NOT to do:
❌ Assuming AI is Neutral – AI learns from biased human data—it’s not automatically fair.
❌ Ignoring Small Sample Sizes – If training data is too narrow, AI won’t generalize fairly.
❌ Letting AI Work Unsupervised – AI should assist hiring, not replace human judgment.
❌ Overcorrecting in One Direction – Fixing bias shouldn’t introduce new discrimination.
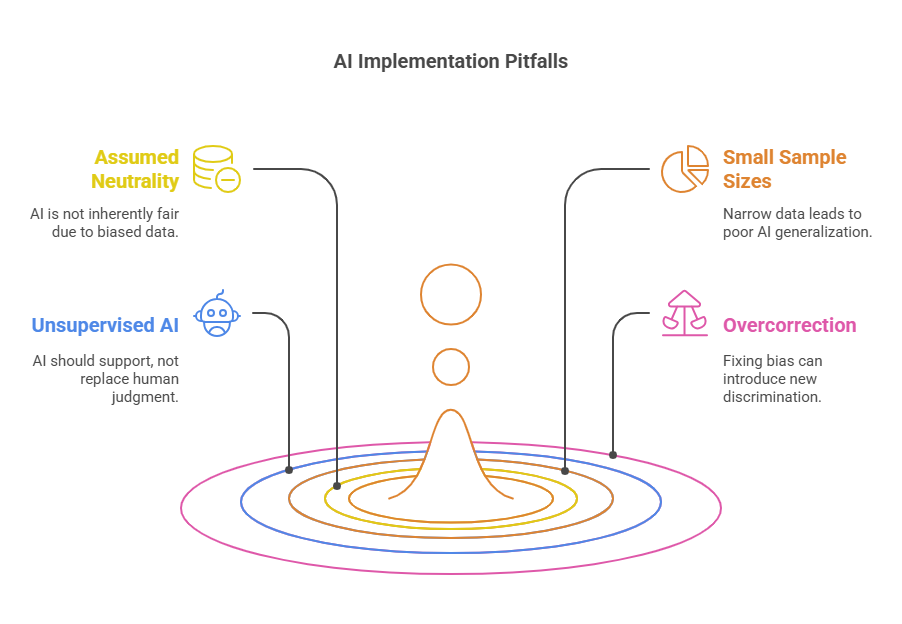
Case Study: "A recruitment AI was adjusted to favor underrepresented candidates—but inadvertently started excluding qualified majority-group candidates. The company had to rework its fairness model."
Final Thoughts: AI is Powerful, But Needs Guardrails
AI in hiring isn’t perfect—but it can be improved. The key to ethical AI recruitment is active oversight, bias audits, and continuous adjustments.
Companies that take proactive steps to detect and fix AI discrimination will build fairer hiring pipelines, enhance diversity outcomes, and strengthen employer trust.
�� Next Up: The Recruiter’s AI Toolkit – 7 Non-Negotiable Skills for 2025 ��
How is your company ensuring AI-driven hiring remains fair and ethical? Drop your thoughts in the comments!